Crafting Culinary Connections: The Power of Two-Tower Embeddings in Revolutionizing Uber Eats Recommendations
- 23 Aug, 2023
- Natural Language Processing
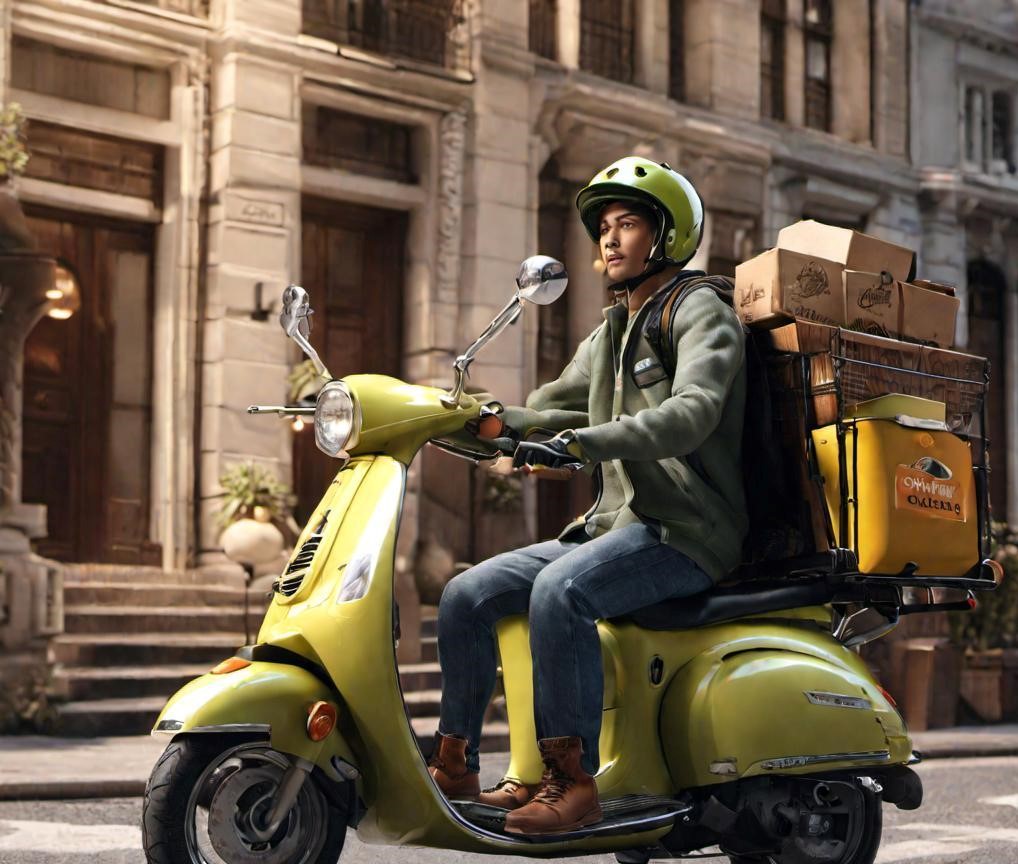
Recommendation systems are essential for helping Uber Eats users like you discover a diverse range of delicious options. From the moment you open the app and explore your feed, the sequence of eateries presented to you is thoughtfully curated by various rankers. These rankers identify the most fitting candidates (restaurants) to display, aiming to make your experience seamless and satisfying. This achievement is powered by an innovative strategy known as Two-Tower Embeddings (TTE), which facilitates efficient customer choices and boosts the system's overall performance.
Elevating Recommendations:
Uber Eats' approach focuses on refining every facet of your experience, starting from the instant you launch the app to view your personalized feed. The arrangement of restaurants in this feed is influenced by various algorithms that strive to showcase the most relevant eateries.
The TTE Model
At the core of this enhancement lies the Two-Tower Embeddings (TTE) model. Visualize two distinct towers—one tower discerns your preferences and search queries (the query tower), while the other grasps the characteristics of different eateries (the item tower). These towers transform information into specialized codes referred to as embeddings. These embeddings facilitate the understanding of your preferences and the attributes of various dining choices.
Crunching Compatibility Scores
In the realm of technology, the Two-Tower Embeddings (TTE) method brings forth a smart concept - compatibility scoring. This process involves multiplying specialized codes from both the query and item towers. The result is a compatibility score that indicates how well your preferences align with available dining options. A higher score means a strong match, suggesting a close fit to your taste. Conversely, a lower score indicates a less suitable match. This compatibility score plays a crucial role in suggesting dining choices that resonate with your palate. It's like tech magic predicting your flavor preferences!
Utilizing Past Insights
The TTE model doesn't just focus on the present—it considers your past interactions too. If you enjoyed a particular eatery before, this information guides the model in comprehending your current preferences. This utilization of historical behavior empowers the model to become increasingly adept at suggesting options that resonate with you.
Scaling Up: From Local to Global
The earlier method faced scalability challenges, especially as Uber Eats expanded to numerous locations. TTE emerged as a solution, simplifying the management of recommendations across various cities. It proved invaluable in handling personalized suggestions, training processes, and accommodating diverse data types.
Overcoming Challenges:
The creation of such a robust system was not without its share of challenges. Managing the model's size and training duration presented significant obstacles. Additionally, traditional evaluation metrics needed adaptation to effectively assess the context-sensitive recommendations offered by the TTE model.
In the domain of recommendation systems, Two-Tower Embeddings have unlocked new dimensions of personalization, scalability, and efficiency for Uber Eats. This inventive approach showcases the fusion of intricate technology and human preferences, culminating in an experience that tantalizes the taste buds of Uber Eats users across the globe.
Powered by Froala Editor