Demystifying RAG: A Technical Deep Dive into AI Enhancement
- 6 Sep, 2023
- Natural Language Processing
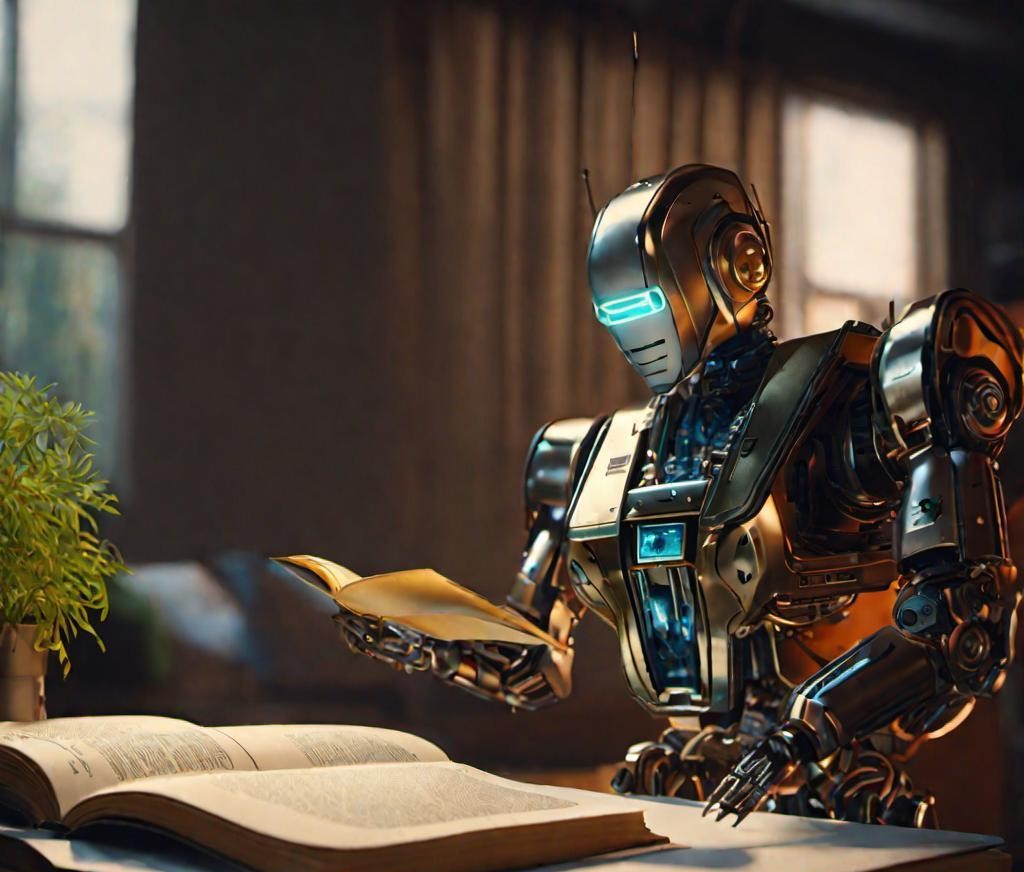
Introduction
In the world of artificial intelligence, there's a game-changing technique called Retrieval-Augmented Generation (RAG). It's like giving AI a superpower to fetch real-time information from the internet or help you to interact with your personal data. In this journey, we'll explore how RAG works, step by step, and talk about the challenges and exciting possibilities it brings.
In the World of Embeddings: Unveiling the Magic of Data Conversion
In our journey into the world of AI and Retrieval-Augmented Generation (RAG), one crucial element is the embedding model. Imagine it as the brain behind the scenes, converting data into numeric vectors—arrays of numbers.
Now, what's the big deal with these vectors? Well, they're like a secret language computers understand. These numerical representations capture the essence or meaning of the input text, much like how another person would understand the spoken words. It's all about enabling computers to search for similar things based on these numeric representations, making it a bridge between humans and machines in the realm of AI.
The Library Organizer - Indexing Knowledge Library
Imagine having a massive library filled with books on various topics. Now, you want to find a specific book quickly, just like you might want precise information from a vast sea of data. This is where indexing comes into play. It's akin to creating a detailed catalog that meticulously lists where each book is located in the library.
Your Data Vault - Pinecone's Role
Now, let's talk about the essential role of tools like Pinecone in this process. Pinecone serves as your trusty guide, similar to a map that helps you navigate the library efficiently. It aids AI in locating the right information swiftly, just as a GPS map on your phone guides you to your destination.
However, like any map, Pinecone needs regular updates. Imagine driving with an outdated GPS map that leads you to the wrong places. Similarly, Pinecone must stay in sync with the ever-evolving landscape of data. Ensuring that it accurately reflects the knowledge repository's contents is vital for it to be a reliable guide in the quest for information.
Hunting for Clues - Retrieving Data
Now, let's dive into the action-packed role of AI in retrieving data. Think of AI as a detective on a mission, much like Sherlock Holmes in a grand library filled with mysteries. When you pose a question to AI, it embarks on a quest for relevant information, just as a detective hunts for vital clues in a labyrinthine library.
AI's goal is to find the best books (or data) that hold the answers you seek. However, like any detective, AI can refine its skills. It can learn to be more precise, agile, and thorough in its search, ensuring that it unearths the most accurate and relevant information for you.
Challenges and Improvements:
1. Perfecting Context Understanding: We need AI to understand context better to reduce mistakes.
2. Keeping the Catalog Updated: Updating the library catalog automatically is a challenge, but it's essential to stay current.
Possible Use Cases:
1. Personalized Education: AI can personalize learning, making it more tailored to each student.
2. Healthcare Advancements: Doctors can use AI to keep up with the latest medical discoveries, which can improve patient care.
3.Chatbot Assistance: From books to user manuals you can now interact with your documents just by creating a chatbot based on you data.
Conclusion
RAG is like giving AI superpowers. It simplifies complex tasks step by step. Challenges exist, but with improvements, RAG promises a future where AI truly understands and serves us better. It's time to make AI work for you!
Powered by Froala Editor