Healthcare and AI
Perhaps the most promising domain for AI applications is Healthcare, given the vast amount of data being generated continuously across hospitals, labs, clinics, etc. Ranging from Computer Vision analysis of various X-Ray/MRI/CT scans, eye/skin images, etc.; Natural Language Processing of patient records; to Signal/Audio/Time-Series analysis of patients' vitals and voice samples - the possibilities are endless. A few such use case examples are:
- Parkinsonism Detection using Smartphone Data: Parkinson’s disease is a neurodegenerative disease that can affect a person’s movement, speech, dexterity, and cognition. Clinicians primarily diagnose Parkinson’s disease by performing a clinical assessment of symptoms. However, misdiagnoses are common. One factor that contributes to misdiagnoses is that the symptoms of Parkinson’s disease may not be prominent at the time the clinical assessment is performed. Machine Learning can help in overcoming these challenges by using long-term data from smartphone-based walking, voice, tapping and memory tests to distinguish between people with and without Parkinson’s disease. (Ref: PhoneMD)
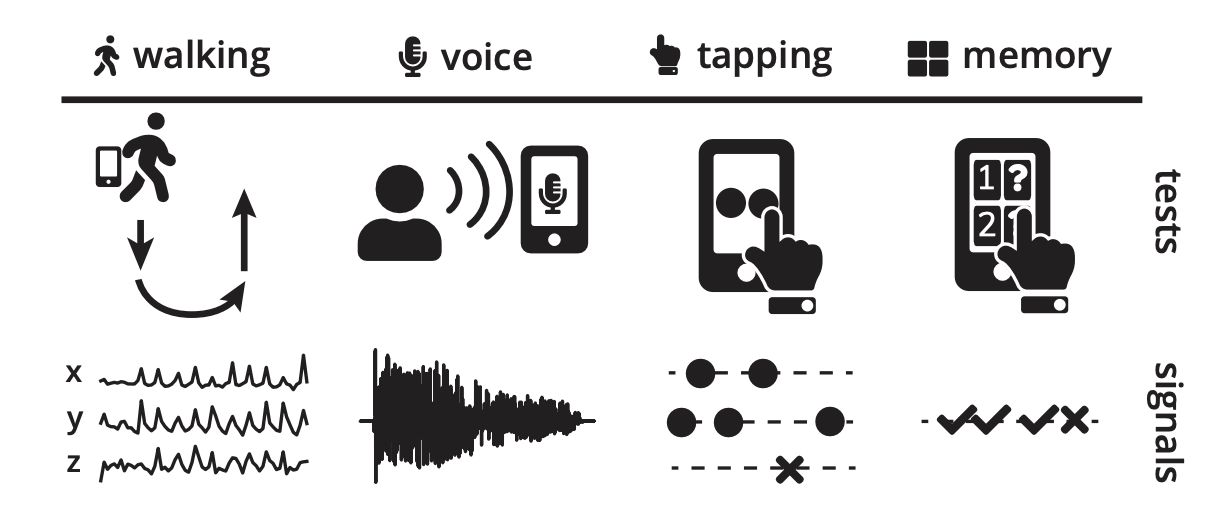
Fig 1: Smartphones can be used to perform tests that are designed to trigger symptoms of Parkinson’s disease (top). During these tests, smartphone sensors record high-resolution signals (bottom) that we can use to distinguish between people with and without Parkinson’s disease. (Ref: PhoneMD)
- Diabetic Retinopathy: Diabetic retinopathy is a diabetes complication that affects the eyes. It's caused by damage to the blood vessels of the light-sensitive tissue at the back of the eye (retina). To study retina, a retinal examination is done where images of retina are taken through fundus photography. Fundus photography involves capturing a photograph of the back of the eye to obtain Fundus images. These are then analyzed by ophthalmologists who look for certain patterns and defects in the image to predict diseases. There are many problems in this system. The world is short of highly qualified ophthalmologists. Due to this people have to wait for long before starting medications. This sometimes worsens the condition. Another crucial disadvantage is a lack of agreement between different doctors on a single profile of fundus. AI can help make diagnosing Diabetic Retinopathy easier, faster and more accessible by accurately interpreting fundus images using Computer Vision.
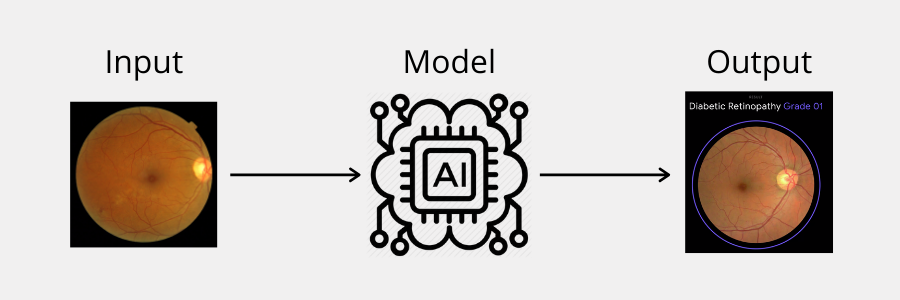
Fig 2: Using Artificial Intelligence to diagnose DR and its severity