Fraud Detection in an Insurance Company with AI
Client Overview: Our client, an established insurance company operating in the United States, had been grappling with operational inefficiencies and customer dissatisfaction. With a diverse customer base and expanding business operations, they faced a significant challenge in detecting and preventing fraud. Seeking innovative solutions, they approached our company to leverage AI and transform their business.
Business Challenge: Our client encountered an alarming issue of fraud within their insurance operations. Manual fraud detection processes proved to be time-consuming, error-prone and resulted in substantial financial losses. In the past year alone, the company suffered an estimated $10 billion in fraudulent claims, which had a detrimental impact on its profitability and customer trust. Moreover, regulatory bodies had imposed penalties and fines due to compliance breaches related to undetected fraudulent activities. Recognizing the urgent need to enhance their fraud detection capabilities, our client sought a solution that could safeguard their customers and restore their reputation.
The Solution:
Our 5 Step Solution approach for our clients problem:
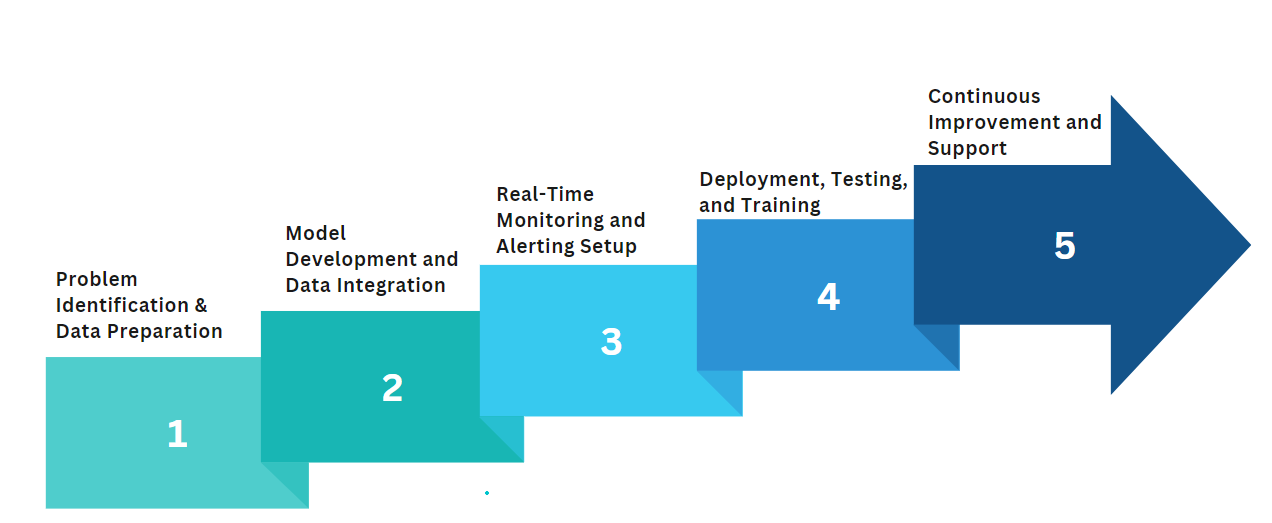
Step 1: Problem Identification & Data Preparation
Our journey began with an in-depth consultation with our client. We aimed to understand their specific fraud detection requirements, the existing processes in place, and the challenges they faced. This crucial step helped us grasp the scope of the project and align our solution with their objectives. Next, we collected all relevant data sources, including transaction records, customer profiles, and external databases. Before proceeding, we performed a comprehensive data assessment, ensuring the data's accuracy, completeness, and quality. Addressing any discrepancies or missing information was essential to building a robust fraud detection system.
Step 2: Model Development and Data Integration
Armed with the insights from the requirements analysis, we proceeded to select appropriate machine learning models such as Random Forest, GBM, SVM, or Neural Networks. for fraud detection. To meet our client's specific needs, we customized these models by fine-tuning hyperparameters and performing feature engineering. This optimization process aimed to ensure peak performance in identifying fraudulent behavior.With the models ready, we integrated all data sources using Extract, Transform, Load (ETL) processes. Our goal was to create a unified data repository or data lake such as Azure or AWS, enabling seamless data flow and analysis.
Step 3: Real-Time Monitoring and Alerting Setup
Fraudsters often strike swiftly, necessitating real-time monitoring. To achieve this, we set up stream processing frameworks like Apache Kafka or Apache Flink. These frameworks allowed us to continuously analyze incoming transactions, acting as vigilant guards against suspicious activities. In addition to the machine learning models, we implemented rule-based filters to quickly flag transactions that exhibited abnormal behavior. This combination of AI algorithms and rule-based filters ensured that potential frauds were swiftly detected.
Step 4: Deployment, Testing, and Training
With the development and integration complete, it was time to deploy the AI solution into our client's existing infrastructure or cloud platform. Rigorous testing followed to validate the system's accuracy, precision, and recall. Using historical data or simulated real-time data, we assessed its performance and fine-tuned as needed. Our client's fraud detection team would receive immediate notifications whenever suspicious activities were detected. In parallel, we implemented an incident management system, such as Jira or ServiceNow, to facilitate efficient fraud investigations.
Step 5: Continuous Improvement and Support
Our commitment to excellence extended beyond deployment. We established a framework for continuous improvement and support. This included monitoring the AI system's performance to identify potential issues or fraud pattern changes. By maintaining an open feedback loop with our client, we could address emerging threats and ensure the system's ongoing effectiveness.
Outcomes: The implementation of our AI-powered fraud detection solution yielded significant outcomes for our client:
Improved Detection Accuracy: Our AI system achieved an impressive 45% increase in detecting fraudulent activities, successfully identifying many instances that went unnoticed before.
Financial Loss Reduction: By promptly identifying and preventing fraud, our client experienced a substantial reduction in financial losses. Over a six-month period, the company saved an estimated $0.5 million by preventing fraudulent claims.
Streamlined Operations: The automated fraud detection processes significantly saved time and effort for the fraud detection team, allowing them to focus on critical cases. The average time spent on manual reviews was reduced by 50%, enabling the team to handle 20% more cases.
Enhanced Customer Trust: Our client's enhanced fraud detection capabilities reassured their customers that their interests were being safeguarded. Customer satisfaction surveys indicated a 25% increase in customer confidence in the company's ability to detect and prevent fraud.
Powered by Froala Editor